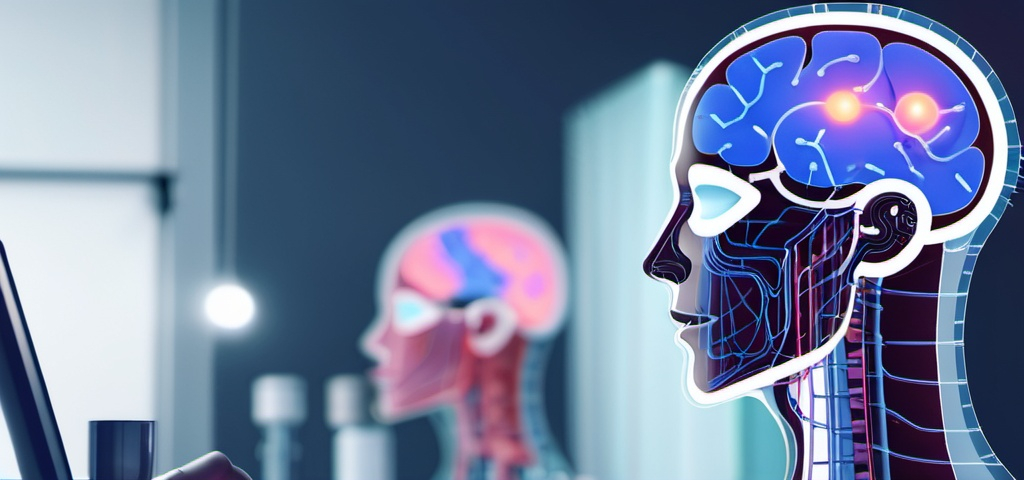
by Mykhailo Mamchur
30 Aug, 2024
The Role of AI in Drug Development: Enhancing Research, Assessment, and Discovery
Introduction
The pharmaceutical industry is constantly evolving, with drug development being one of its most dynamic and challenging areas. Traditionally, this process has been lengthy, complex, and costly, with high risks and uncertainties. However, the integration of artificial intelligence (AI) into drug development is transforming the landscape. AI’s ability to analyze vast datasets, predict outcomes, and streamline processes is accelerating the pace of drug discovery, enhancing the precision of predictions, and significantly reducing development costs.
By automating complex tasks and providing deeper insights, AI enables more effective treatments to reach the market faster. Artificial Intelligence not only improves drug discovery directly, but it also helps with research along the way.
Steps of Drug Discovery
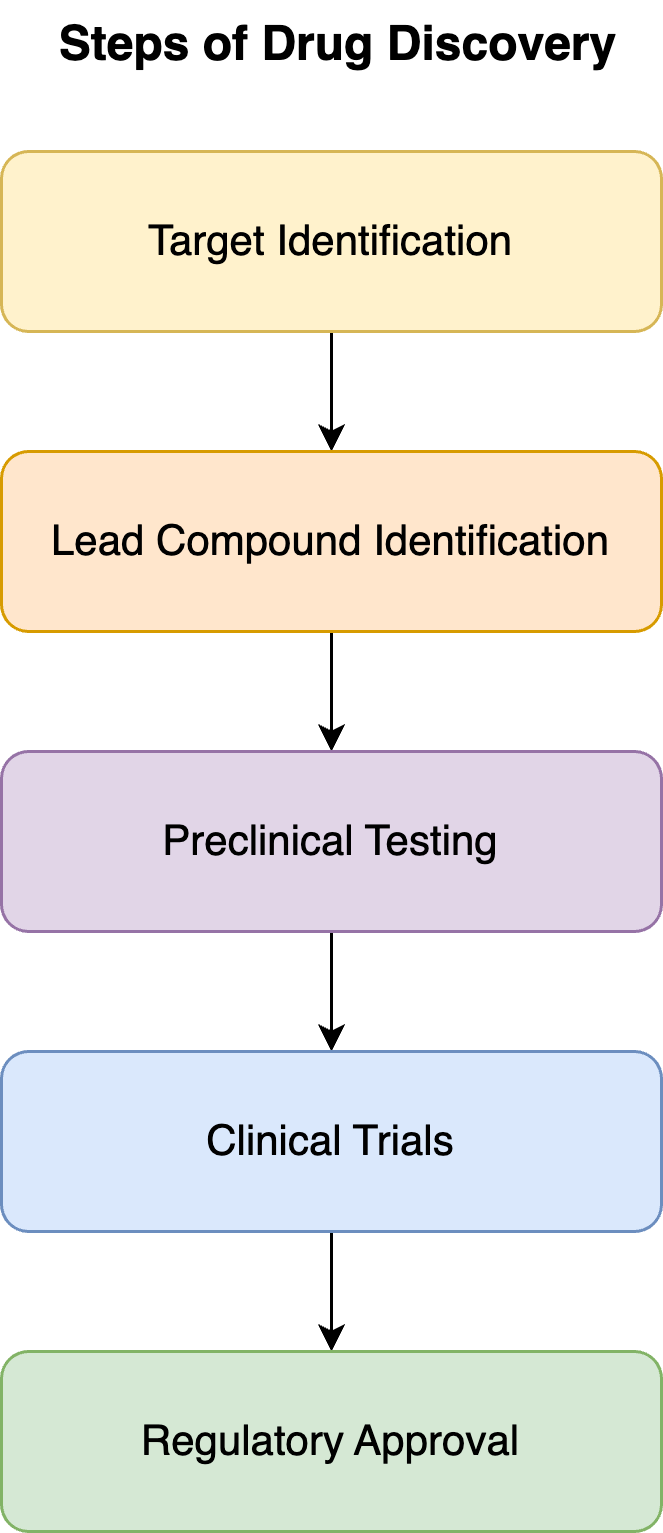
The drug discovery process is a multi-step journey that involves rigorous research, testing, and validation. The key stages include:
- Target Identification: Identifying the biological target associated with a disease (e.g. type 2 diabetes/obesity disease and glucagon-like peptide-1 receptor target).
- Lead Compound Identification: Discovering a chemical compound that interacts with the target (e.g. semaglutide).
- Lead Compound Assessment and Optimization: Scoring the lead compound and refining it to enhance its efficacy and minimize toxicity.
- Preclinical Testing: Conducting lab-based studies to evaluate the safety and efficacy of the compound.
- Clinical Trials: Testing the drug in humans to ensure its safety and effectiveness across multiple phases.
- Regulatory Approval: Submitting the drug for approval from regulatory bodies before it can be marketed.
Each of these steps in the drug discovery process requires rigorous data collection, thorough analysis, and precise interpretation to ensure successful outcomes. Traditionally, these tasks were handled manually, often leading to significant delays and potential errors. However, with the use of AI, we are now able to automate and streamline critical aspects of this process.
Specifically, AI can also play a pivotal role in enhancing the efficiency and accuracy of compound stability assessments and toxicity evaluations, such as hERG blocker classification—two crucial steps that can significantly impact the speed and success of drug development.
Enhancing Compound Stability Assessment with AI
In the drug discovery process, assessing the metabolic stability of a compound is crucial. Metabolic stability refers to how long a drug remains intact before it is metabolized by the body, which directly impacts its efficacy and safety. Understanding the stability of a compound is vital in drug development because it directly impacts how long a drug will be effective in the body.
Traditionally, this assessment involves complex laboratory procedures and the analysis of large datasets to determine the compound’s stability under various conditions.
Our AI-powered Metabolic Stability Estimator simplifies this assessment. By analyzing the chemical structure of a compound, it quickly predicts whether a drug candidate is likely to remain stable under various conditions or degrade too quickly.
The business value here is clear: faster and more reliable stability assessments mean you can move forward with promising drug candidates more quickly, saving valuable time and resources in the development process.
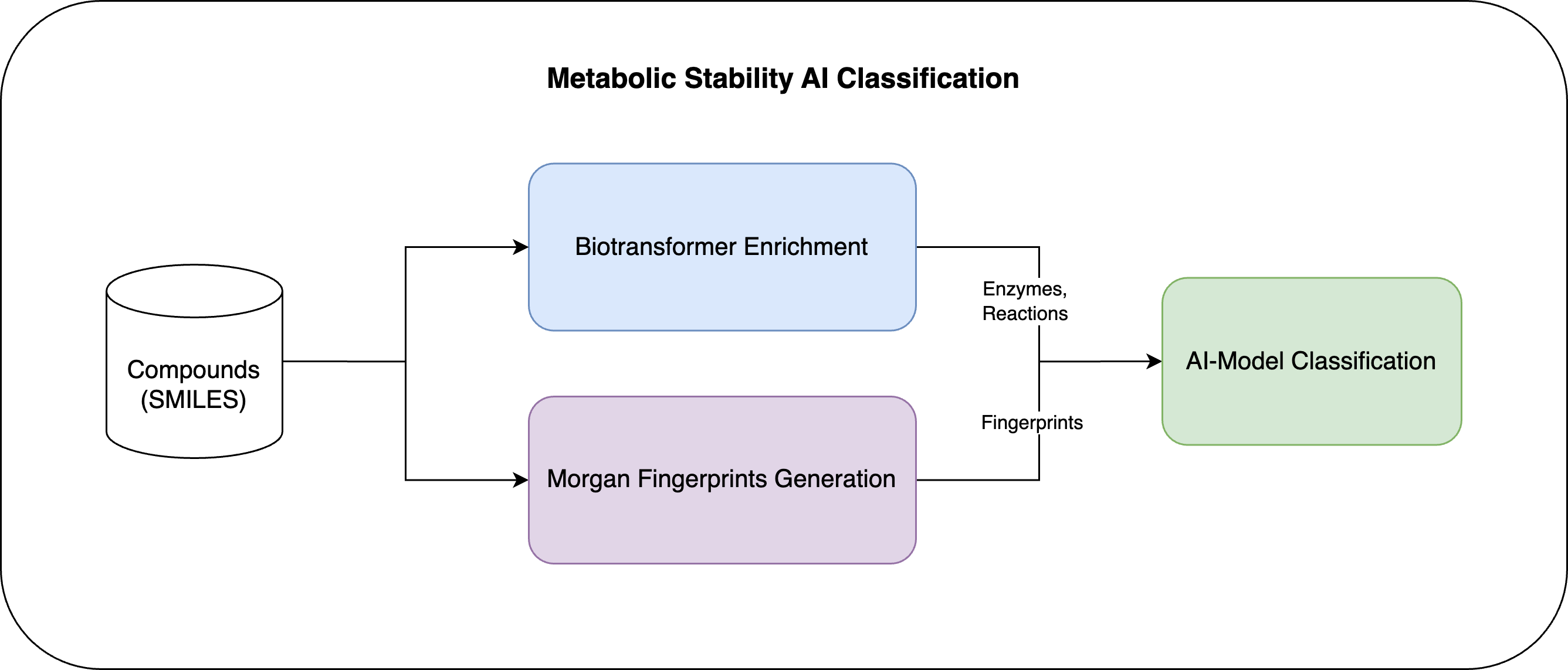
From a technical standpoint, our AI-powered Metabolic Stability Estimator works by first computing enzymes, reactions, and fingerprints for the compound utilizing the Biotransformer toolkit and Morgan Fingerprint algorithm. These features are then combined and transformed to serve as input to the AI model for predicting metabolic stability.
Enhancing Compound Toxicity Assessment with AI
One of the biggest concerns in drug development is ensuring that a new drug won’t cause harmful side effects, particularly those related to heart health. Specifically, it’s crucial to determine whether a compound might interfere with the heart’s electrical activity, which is often assessed through hERG (human Ether-a-go-go-Related Gene) testing. Compounds that block the hERG channel can lead to serious cardiac side effects, such as arrhythmias, making this a critical checkpoint in the drug development process.
Traditionally, hERG toxicity is assessed using a combination of laboratory testing and computational modeling. In the lab, researchers use patch-clamp assays, which are highly specialized experiments that measure the ionic currents in heart cells. This method, while accurate, is both time-consuming and costly. It requires access to expensive equipment, skilled technicians, and extended periods of time to obtain results.
Our AI-powered hERG Blocker Classifier addresses these challenges head-on. By leveraging artificial intelligence algorithms, the tool quickly analyzes a compound’s chemical structure and predicts its potential to block the hERG channel. This AI tool is built on large datasets that include both toxic and non-toxic compounds, enabling it to make accurate predictions even with novel compounds that haven’t been extensively studied before.
The ability to make such assessments quickly and accurately means that your development team can focus resources on the most promising candidates, thereby increasing the likelihood of success in later stages of development.
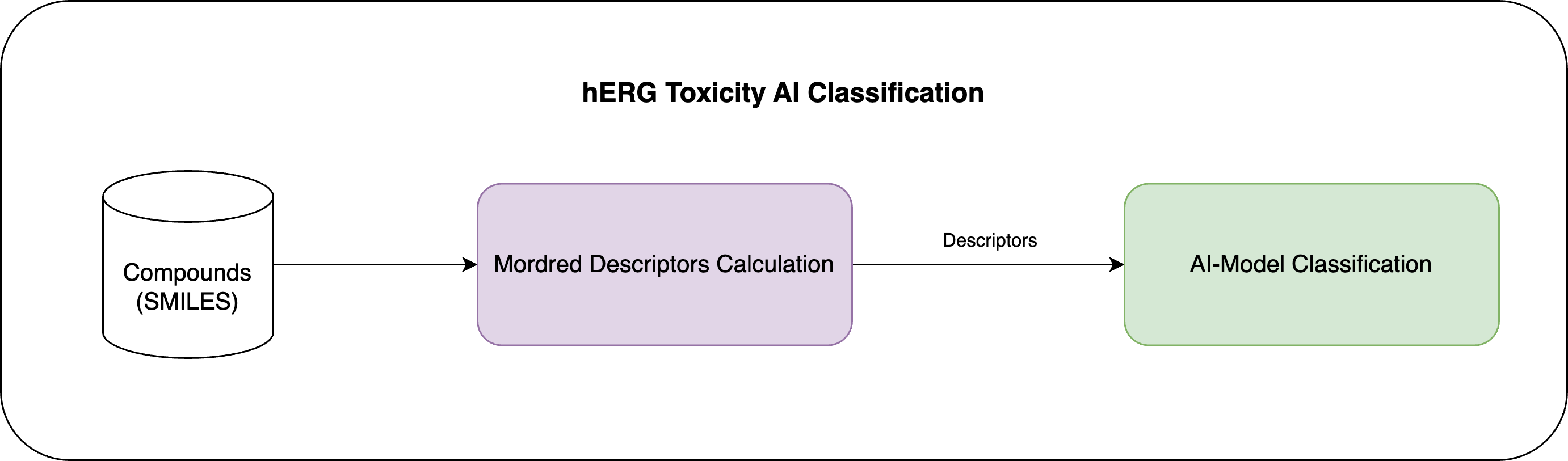
From a technical standpoint, our AI-powered hERG Blocker Classifier works by first computing Mordred Descriptors for the compound. These features are then transformed to serve as input to the AI model for predicting hERG toxicity assessment.
Conclusion
Integrating AI into drug discovery is not just a technological upgrade – it’s a strategic move to enhance efficiency, accuracy, and speed in developing new treatments. By automating tasks like metabolic stability and hERG toxicity assessments, AI tools significantly reduce the time and resources needed to bring a drug to market.
Key Benefits
- Metabolic Stability Estimator:
- Time Reduction: Cuts assessment time from weeks to hours.
- Cost Efficiency: Lowers expenses by reducing lab work.
- Faster Decisions: Enables quicker, more informed choices.
- hERG Blocker Classifier:
- Speed: Reduces toxicity classification from weeks to hours.
- Precision: Delivers reliable early-stage insights.
- Cost Savings: Automates and lowers assessment costs.
Adopting AI in your drug discovery pipeline gives you a crucial edge in a competitive industry, ensuring your R&D is both innovative and cost-effective. As the pharmaceutical industry evolves, integrating AI is essential for bringing life-saving drugs to market faster and more efficiently.
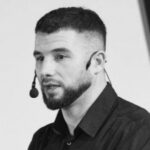
Alex Gurbych
Chief Solutions Architect
Receive a professional and in-depth consultation from an experienced expert. Get tailored advice to address your specific needs and achieve your goals effectively.