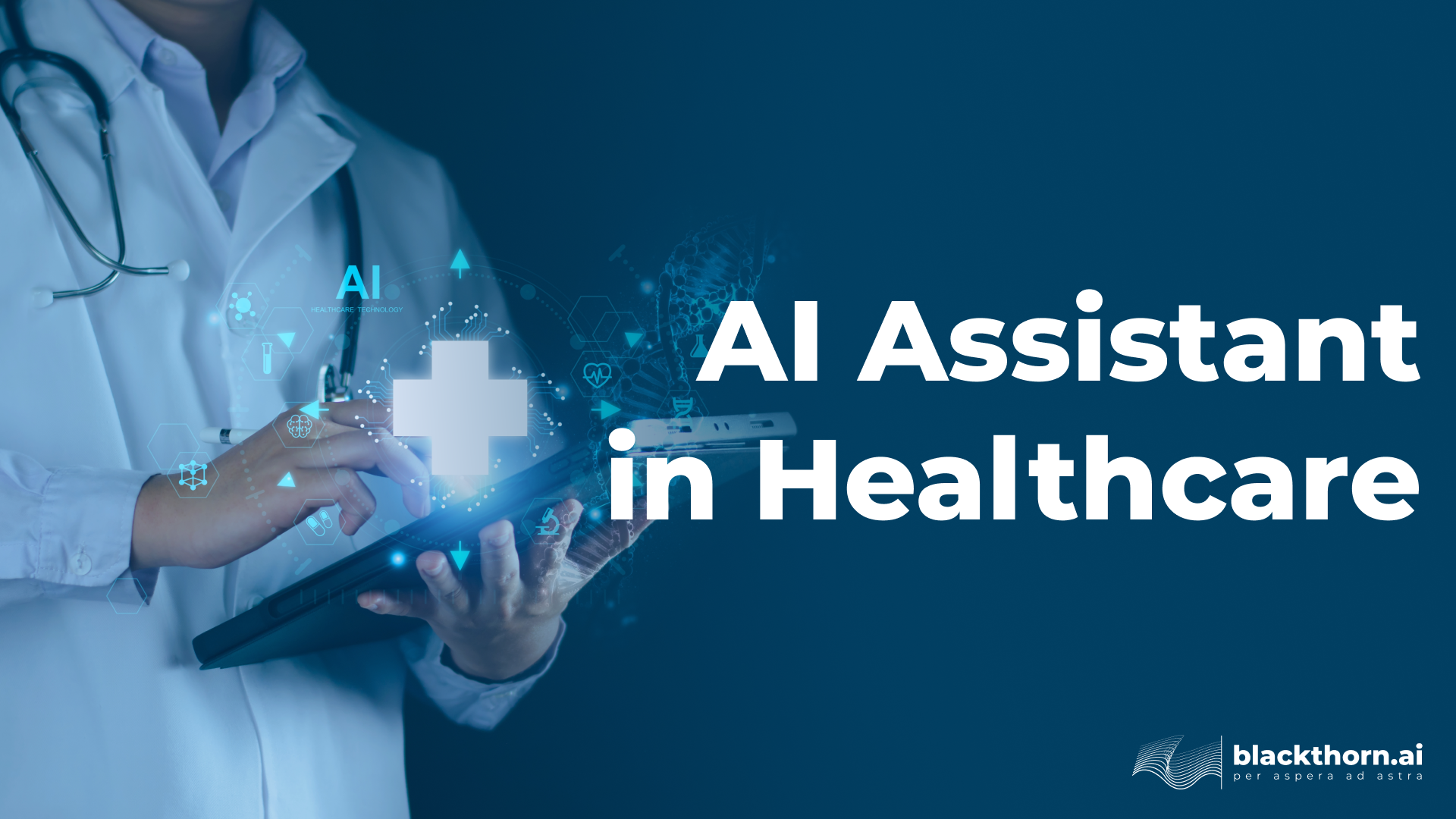
by Danylo Melnyk
12 Apr, 2024
How can you leverage LLMs in healthcare to boost your company performance?
1. Introduction to Large Language Models (LLMs) in Healthcare
Large language models (LLMs) are advanced artificial intelligence systems that can analyze huge amounts of text, allowing them to understand, manipulate, and even generate human language.
The world was surprised by the latest advances in the development of artificial intelligence. ChatGPT along with other models from companies like Meta AI, Google DeepMind, and Mistral AI highlighted the unique ability of large language models to understand, generate, and interact with natural human language.
While chatbots are obvious applications of LLM, they can be used for various natural language processing (NLP) tasks:
- Text classification
- Extraction of required information from huge texts
- Question answering
- Content creation
- Text summarizing
- Machine translation
The revolutionary power of LLM is that they can learn how to solve new tasks even from a few examples Language Models are Few-Shot Learners.
Overall, LLMs are a powerful tool that can be applied to any industry requiring a good understanding of complex datasets, such as healthcare. Large language models in the healthcare sector are utilized to enhance patient care, streamline administrative tasks, and accelerate medical research. They have a role in personalized medicine, allowing patient-specific treatment plans.
Understanding and competent usage of LLMs is crucial for healthcare organizations seeking to deliver exceptional patient care in the modern era.
2. The Current State of Healthcare and Technological Needs
Currently, healthcare faces challenges in the way of high-quality service delivery. Among these challenges:
- Poor communication between healthcare providers, which often leads to misdiagnoses and ineffective treatment plans;
- Lack of resources, limiting access to care for many patients;
- Staff burnout caused by overwork and the stressful nature of healthcare which leads to decreased job satisfaction and a decline in patient care quality;
- High research costs.
- Demand for personalized patient care is rising, patients are seeking the most effective treatments for their unique needs and circumstances.
Technological advancements, particularly the usage of large language models, offer promising solutions for these issues. Leveraging large language models can enhance communication by providing tools for better patient-provider interactions and streamlining information transfer. They can assist during the education and training of medical professionals identifying gaps in care and opportunities for improvement. By automating administrative tasks, LLMs can prevent staff burnout, allowing healthcare professionals to focus more on patient care. They can assist in research by analyzing vast amounts of medical literature. This can aid researchers, clinicians, and medical professionals in finding the required information, and verifying their decisions against known studies. Additionally, LLMs can help develop personalized care plans by analyzing patient data and generating insights tailored to individual health profiles. Language models could monitor the care process, and analyze customer feedback for early detection of potential problems.
Leveraging large language models in healthcare is not just a possibility but a necessity. LLMs are providing the way for a future where high-quality care is accessible, equitable, and adapted to the needs of each patient. In the next sections, we will describe the landscape of large language models in medicine.
3. How LLMs Are Transforming Healthcare: A General Overview
Large language models have the potential to significantly change healthcare sector. Their unique ability to organize, extract, analyze, and interpret enormous amounts of unstructured data can greatly improve healthcare data management. This includes summarizing complex medical documents, like patient records, research papers, and clinical records into short but informative views, making them more accessible and usable for healthcare professionals.
Large language models could also lead to enhanced patient-provider communications, especially in multilingual environments. These models can ease more personalized, and engaging interactions, contributing to an enhanced healthcare experience.
In addition, LLMs can support decision-making processes by providing comprehensive insights for doctors. They can help doctors find similar cases to make evidence-based recommendations.
What are medical large language models? Medical or healthcare large language models are built based on general LLM but with additional training (fine-tuning) on vast amounts of medical literature, patient records, clinical guidelines, and other healthcare-related data. This allows them to be adapted to the domain-specific language of healthcare and better understand medical terminology.
The use of specialized LLMs benefits in several ways:
- More accurate and relevant insights for clinical decision-making;
- Improved efficiency of healthcare services by understanding and processing healthcare-specific data and inquiries;
- Production of more accurate, relevant, and evidence-based answers.
The adoption of large language models accelerating healthcare businesses today. The use of medical large language models has the potential to revolutionize healthcare, improving care quality, streamlining workflows, and optimizing resource allocation. Utilizing the power of LLMs, healthcare businesses can step into a new era of efficiency, accuracy, and enhanced patient experience.
4. Detailed Use Cases of LLMs in Healthcare
Large language models are transforming the healthcare sector.
These models are considered foundational due to their broad training on diverse data sets and can be applied to a wide range of downstream tasks in any domain. Production-grade LLMs are categorized into 2 groups: encoder-only and decoder-only.
Encoder-only models, like BioBERT, ClinicalBERT, and BlueBERT are focused on understanding or classifying input data.
Decoder-only models with GPTs, LLaMA, Mistral, Gemini, and Claude are known for their ability to generate text. Finetuned versions of these models, such as MedAlpaca, MedPalm-2, BioGPT, Meditron, and BioMistral offer a better understanding of the healthcare domain.
The following table provides a diverse list of applications for LLMs in healthcare.
Healthcare Aspect | Applications of LLMs |
Healthcare Communication | – Help in multilingual interactions and translate medical terms. – Improve messages’ emotional coloring. – Improve patient engagement, making conversations more understandable and engaging. |
Healthcare Data Management | – Streamline searching, and summarizing of large texts. – Simplify data mining: find the required information, mark named entities, and classify texts. – Assist in SQL database management: transform natural language into SQL queries, help refine or explain complex queries. |
Administrative Tasks | – Guide patients to needed departments. – Generate reports from text like visit notes, patient records, etc. – Automate appointment scheduling, check-in, and other administrative procedures. |
Claims Management | – Validate insurance claims, and extract key details. – Detect fraud and spot inconsistencies. |
Clinical Decision Support | – Provide personalized patient insights. – Retrieve literature with similar cases. – Assist in treatment prioritization, and optimize treatment plans. |
Medical Research | – Generate new ideas. – Automate literature reviews, and summarize papers. – Identify gaps, and find required literature. – Anonymize the data. |
Education and Training | – Identify gaps in knowledge, and provide individual learning paths. – Simulate patient cases. – Help in onboarding by providing answers using internal documentation. |
Personalized Care | – Analyze patient records, lifestyle, and preferences to produce personal care plans and treatments. – Give personalized lifestyle advice and health recommendations. |
Monitoring | – Monitor patient’s feedback. Determine the sentiment of the text. – Check patient’s records, and find errors. |
After demonstrating various ways LLMs are used in healthcare, let’s focus on three areas:
Healthcare Communication: language models assist in multilingual healthcare environments. Models trained on multilingual medical data can help translate messages, especially medical terms, erasing language barriers. Decoder-only models could enhance messages’ emotional tone, making professional texts more empathic. They could improve patient engagement, making conversations more understandable and interesting.
Healthcare Data Management: BERT-based models can be used for data mining: marking named entities, and classifying texts. Decoder-only models could streamline searching, and summarizing large texts. They could extract required fields from unstructured documents. Models like SQLCoder can ease work with SQL databases: generating SQL queries from natural language requirements, validating, refining, and optimizing existing ones, or just explaining complex queries.
Large language models in claims management could help validate insurance claims and extract key information LLMs can streamline the claims process.
Choosing the right language models in healthcare domain depends on your requirements. The LLM field is evolving, with new and more specialized models presented monthly. It’s essential to pick a model that fits your needs, considering licensing, data privacy, model speed, and costs. Working with a partner with deep knowledge and experience in the machine learning field and large language models is crucial for the delivery of a high-value LLM-powered system.
5. The Benefits of Implementing LLMs in Healthcare Systems
Integrating large language models into healthcare could provide multiple strategic benefits for your business.
LLMs allow the provision of personalized patient care, analyzing patients’ medical histories, preferences, and vitals providing doctors with individual insights. This allows doctors to create personalized treatment plans.
LLMs can significantly improve operational efficiency within the healthcare sector. By automating and optimizing routine tasks like appointment scheduling, filling visit notes, and report generation, they allow professionals to focus on patient needs. The application of LLMs in healthcare can optimize processes to reduce errors and improve quality of care at your facility.
LLMs provide transparency and flexibility simplifying complex medical information for patients and their families, adapting to their knowledge and morale.
LLMs could be powerful research assistants, automating literature reviews, and summarizing papers. They can process vast amounts of medical journals, clinical trials, and other professional text sources.
LLMs can help in training new workers helping them to familiarize themselves with internal documents, protocols, and procedures.
Usage of large language models in medicine has the potential to improve access to health care. They can be used during remote consultations. LLMs can analyze patient data to streamline triage processes, helping patients to receive appropriate levels of medical care. Usage of LLMs during insurance claims management may speed up the process and prevent abuse.
6. Challenges and Considerations for the Future of LLMs in Healthcare
While LLM adoption promises great changes in healthcare, their implementation requires careful consideration. Here, we explore some key challenges:
Data Security and Privacy
Patient data security and privacy have always been essential for healthcare. The development of an LLM-based system should include robust security measures to ensure patient data confidentiality and compliance with strict privacy regulations like GDPR and HIPAA.
Compliance and Regulatory Hurdles
Considering legal regulations from the start is crucial for healthcare artificial intelligence software development. With evolving laws like the EU’s AI Act, balancing innovation with compliance becomes necessary.
Ethical Use and Bias
LLMs, similarly to other artificial intelligence systems, may inherit unwanted patterns from the training data. This could lead to biases, such as unequal treatment, reinforcing stereotypes, and incorrect assumptions. In addition, significant risks come from adversarial attacks, where inputs are manipulated to generate misleading outputs or disclose private info.
Computational Resources and Expertise Requirements
Developing and running LLMs require significant computational resources and expertise. Choosing the right large language model has an impact not only on system capabilities, accuracy, and reliability but also on computational requirements, leading to inference costs and latencies.
Strategic Integration and Ongoing Support
Often health care workers are quite conservative and have concerns about adopting new technologies. LLMs must be properly integrated with existing healthcare IT systems and workflows to minimize staff disruption.
Continuous support is essential because constantly evolving healthcare environment and LLM technologies.
Blackthorn.ai is an AI software development company at the forefront of this exciting revolution. Our company could not only develop end-to-end solutions but also help you with AI/ML integration into your existing products. Our projects included pioneering solutions for treatment optimization, administrative efficiency tools, and the application of computer vision models to improve diagnostic accuracy. We could use our extensive experience working with real-world healthcare problems to build great LLM-powered software.
7. Conclusion: The Future Prospects of LLMs in Healthcare
Large language models’ ability to process huge datasets and enhance human-language interaction are already influencing the field of healthcare today. The ability to understand complex medical information, and use knowledge from large amounts of training data combined with a capacity for reasoning and clear communication are the power of medical large language models.
The capacity of large language models is set to grow exponentially
The development of LLM-powered AI agents is one of the most promising perspectives for future improvements. These systems could interact with external tools. For example, it may request to obtain results from web searches, download web pages, and use newly obtained information to complete the task. They could send emails, create calendar entities, etc. Tools in LLM’s arsenal could be even more powerful: mathematical engines, domain-specific computational tools, or even code execution. They can create and execute queries to databases, and write computer programs for specific tasks. These improvements may allow LLM to solve problems more precisely.
The future of large language models in medicine extends beyond text data. The development of multimodal language models allows for incorporating not only text but other information types: images, audio, or video for a more complete understanding of medical situations.
By unlocking these advanced capabilities, LLMs have the potential to find more ways to improve care quality.
Successful implementation of the LLM system requires a strategic approach. Factors such as data protection, legal compliance, bias mitigation, and efficient use of computing resources need to be proactively addressed.
Selecting a competent technological partner is an essential decision for healthcare business success in the modern world. Our pioneering work at Blackthorn.ai, leveraging different machine learning techniques, including LLMs usage could help us to be at the cutting edge of AI progress in the healthcare sector.
FAQs
- What are Large Language Models (LLMs)?
LLMs are advanced AI systems trained to understand, reason, and interact using human language on large datasets from a variety of sources. They can perform a wide range of tasks, such as translation, content creation, and answering complex questions. - How are LLMs used in healthcare?
What is the importance of medical large language models? In healthcare, LLMs could be used to improve patient care and administrative efficiency, speed up medical research, and much more. Medical large language models are especially fine-tuned on healthcare data, allowing a better understanding of medical language. - What to consider during partner selection for LLM system implementation?
Consider partner expertise in artificial intelligence and machine learning, especially in healthcare applications. Search for experience in successfully implementing complex systems. Ensure that the partner understands the unique challenges of healthcare data regulatory compliance (such as GDPR and HIPAA) and can deliver a system that meets your specific needs while being scalable, efficient, and cost-effective.
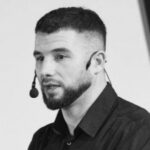
Alex Gurbych
Chief Solutions Architect
Receive a professional and in-depth consultation from an experienced expert. Get tailored advice to address your specific needs and achieve your goals effectively.