Generation of drug molecules with desired properties
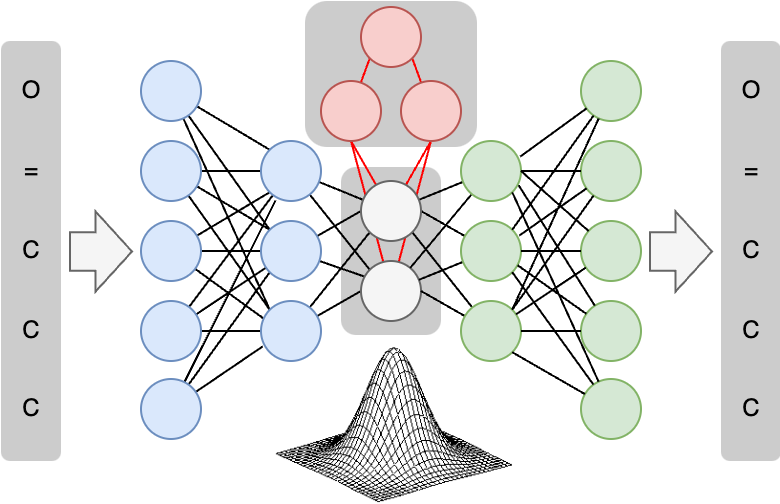
Business Goals
- Generate and verify the chemical correctness of novel drug candidates with desired properties
Results
- The application outputs novel and chemically correct drug-candidates in seconds and estimates their biological and physicochemical properties without conducting wet-lab tests.
Implementation Details
- Several deep neural networks were combined to map discrete molecular representations into continuous distribution. Later, novel drug candidates with desired properties are generated from the retained latent space.
- Molecular generation is performed either by fully-connected feed-forward or by seq-to-seq recurrent neural network with added heads for properties control.
- Subsequently, the generated structures are checked for chemical correctness and rectified, if needed.
- Finally, a report is automatically built for the final outcomes. Physicochemical, biological, structural, and other features of the designed molecules are presented in the report in a human-friendly form.
Industry
Service
Keywords
- Pharmaceuticals
- Drug Discovery
Roadmap
/*=
$user_is_authed
? declense_numeral(get_field('duration'), 'month', 'months')
: 'X months';
*/ ?>
Literature review, AI technology design, and PoC (1 month)
Core AI technology development (3 months)
Generated drug candidates report development (1 month)
Deployment, integration, and productionalization (2 months)
Sign up to receive the project description
Want to talk?
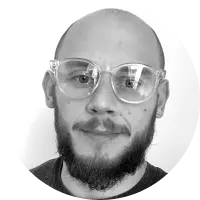
Michael Gurbych
Director,
Operations and Finance
Operations and Finance
Roadmap
/*=
$user_is_authed
? declense_numeral(get_field('duration'), 'month', 'months')
: 'X months';
*/ ?>
Literature review, AI technology design, and PoC (1 month)
Core AI technology development (3 months)
Generated drug candidates report development (1 month)
Deployment, integration, and productionalization (2 months)