Chemical Sciences
Genome Analysis Data Platform
A middle-size pharmaceutical company focused on the development and commercialization of innovative therapies and drugs for rare genetic disorders.
Business Goals
- Develop a large-scale whole-genome genotyping and annotation platform.
- Achieve end-to-end processing of one sample in hours instead of ordinary days and weeks.
- Ensure under 2 seconds latency for analytical requests.
Challenge
- Hundreds of TB of data to ingest, transform, store, and analyze.
- Tens of conditional steps of the workflow to be managed by the workflow itself.
- Parallel execution, management, and monitoring of hundreds of high-load computational tasks.
- High autoscaling demands due to spiky loads.
- Fast analytical queries in SQL format over hundreds of GB of semi-structured genomic data.
- Cost-effective and secure storage of hundreds of TB of data.
- User-friendly web interface for scientists.
Results
- The data platform for rapid WGS genotyping, annotation and analysis was delivered.
- Reads of thousands of patients have been collected, preprocessed, stored, and analyzed.
- Correlation of genetic factors with disease expression and drug response was studied and contributed to the development of vaccines (under NDA).
Implementation Details
- Raw reads generated from Illumina sequencers were uploaded to the object storage and processed in a multi-step workflow to identify the variation in a biological sample compared to a standard genome reference. The resulting variants were combined with other information to identify genomic variants highly correlated with the disease and drug response.
- The workflow included such steps as variant calling (Deep Variant), genome annotation (VEP and LOFTEE), variant classification (CADD and DANN deep learning extension), and phenotype-to-genotype correspondence.
- Apache Airflow was used for the authoring, scheduling, and monitoring of the workflows. Computational genetics algorithms and environments were containerized (Docker) and pushed to a cloud container registry. Airflow runs a separate compute environment with GPU for each task, monitors the execution status, and automatically collapses the environment as soon as the task is completed.
- Raw WGS reads (FASTQ) were transformed into structured (VCF, annotated VCF) and loaded into the columnar OLAP data warehouse.
- Lifecycle policies were implemented for automatic separation of the artifacts into “hot” (used in analytics) and “cold” (not used currently, but may be needed for audit).
- The web portal was designed, implemented, and deployed to the serverless auto-scalable cloud compute engine.
Get a technical consultation
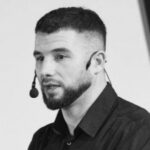
Alex Gurbych
Chief Solutions Architect
Receive a professional and in-depth consultation from an experienced expert. Get tailored advice to address your specific needs and achieve your goals effectively.