Defence
Landmine Detection using Deep Learning and Drones
Automated detection of mines and missile remnants using drones equipped with multispectral sensors
Business Goals
The primary objective was to develop a fast, safe, accurate, remote system for detecting landmines, reducing the risks associated with manual detection and increasing the efficiency and coverage of demining operations.
Challenge
Landmines pose significant threats in post-conflict regions, resulting in numerous casualties and impeding socio-economic development. Traditional demining methods are labour-intensive, dangerous, and often imprecise, requiring an innovative and efficient solution to address this grave issue.
Results
- 22% Improvement in Detection Accuracy
The fine-tuned automated drone system demonstrated 92% average accuracy, reflecting a 22% improvement in correctly identifying landmines over the manual method with 70% accuracy. - 20-Fold Increase in Detection Speed
The automated system surveys up to 10,000 square meters per day, resulting in a 20-fold increase over the manual method with approximately 500 square meters per day. - 90% Decrease in Incidents
The AI drone system reduced the accident rate to 0.5 accidents per 1,000 operations, marking a 90% decrease in incidents compared to the manual landmine surveys with average of 5 accidents per 1,000 operations. - 80% Cost Reduction
Mine detection costs dropped to $1 per square meter, signifying an 80% cost reduction as opposed to the manual method costs of around $5 per square meter surveyed - 6-Day Reduction in Reporting Time
The automated system requires only 24 hours to analyze and report findings for a specific area, while the manual one takes about 7 days for the same process, showing a 6-day reduction in reporting time. - 83% Decrease in Environmental Disruption
The drone AI system reduced environmental disturbance to 5% comparing to the manual approach with approximately 30% of the surveyed area’s vegetation disruption, marking an 83% decrease. - 80% Reduction in Training Effort
Manual Method: 200 hours of specialized training required for deminers
The automated system required on average 40 hours of training for drone operators and system maintenance, indicating an 80% reduction in training hours. - 2x Easier Scaling
Doubling AI drone detection operations require only a 50% increase in resources, while doubling manual operations require doubling personnel and resources.
Implementation Details
- Preliminary Research and Data Collection – 4 weeks
- Conducted a comprehensive review of existing landmine detection methods and their limitations.
- Gathered a preliminary dataset of aerial images highlighting anti-infantry and anti-vehicle landmines, locations, terrains, and false positives from various sources and contaminated areas.
- Identified specific limitations of using DJI drones and the most represented types of mines.
- Data Augmentation and Baseline Model – 4 weeks
- Expanded the dataset using augmentation techniques to ensure a robust training set, extending set of terrains and environmental conditions.
- Designed the baseline computer vision model architecture, focusing on convolutional neural networks (CNN) suitable for landmine detection tasks
- Performed hyperparameter tuning and trained the baseline CNN model.
- Drone Integration and Data Acquisition – 8 weeks
- Integrated the DJI drone with the mine detection software system.
- Established a data pipeline to process drone-captured images in real-time.
- Conducted field tests to collect real-time aerial data from multiple terrains.
- Mine Detection Model Optimization – 8 weeks
- Used the enhanced dataset to train the improved computer vision model.
- Iteratively optimized the model based on the cross-validation dataset performance, fine-tuning hyperparameters and revising the model architecture.
- Achieved significant improvements in real-world mine detection accuracy on multiple terrains and weather conditions.
- Final Deployment and Field Testing – 6 weeks
- Implemented the feedback loop to allow for real-time model adjustments based on field results.
- Integrated DJI drone and updated computer vision system.
- Conducted extensive field testing in real-world scenarios, assessing the model’s performance in varied terrains and conditions.
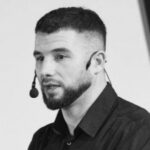
Alex Gurbych
Chief Solutions Architect
Receive a professional and in-depth consultation from an experienced expert. Get tailored advice to address your specific needs and achieve your goals effectively.