Molecular Binding Affinity Prediction
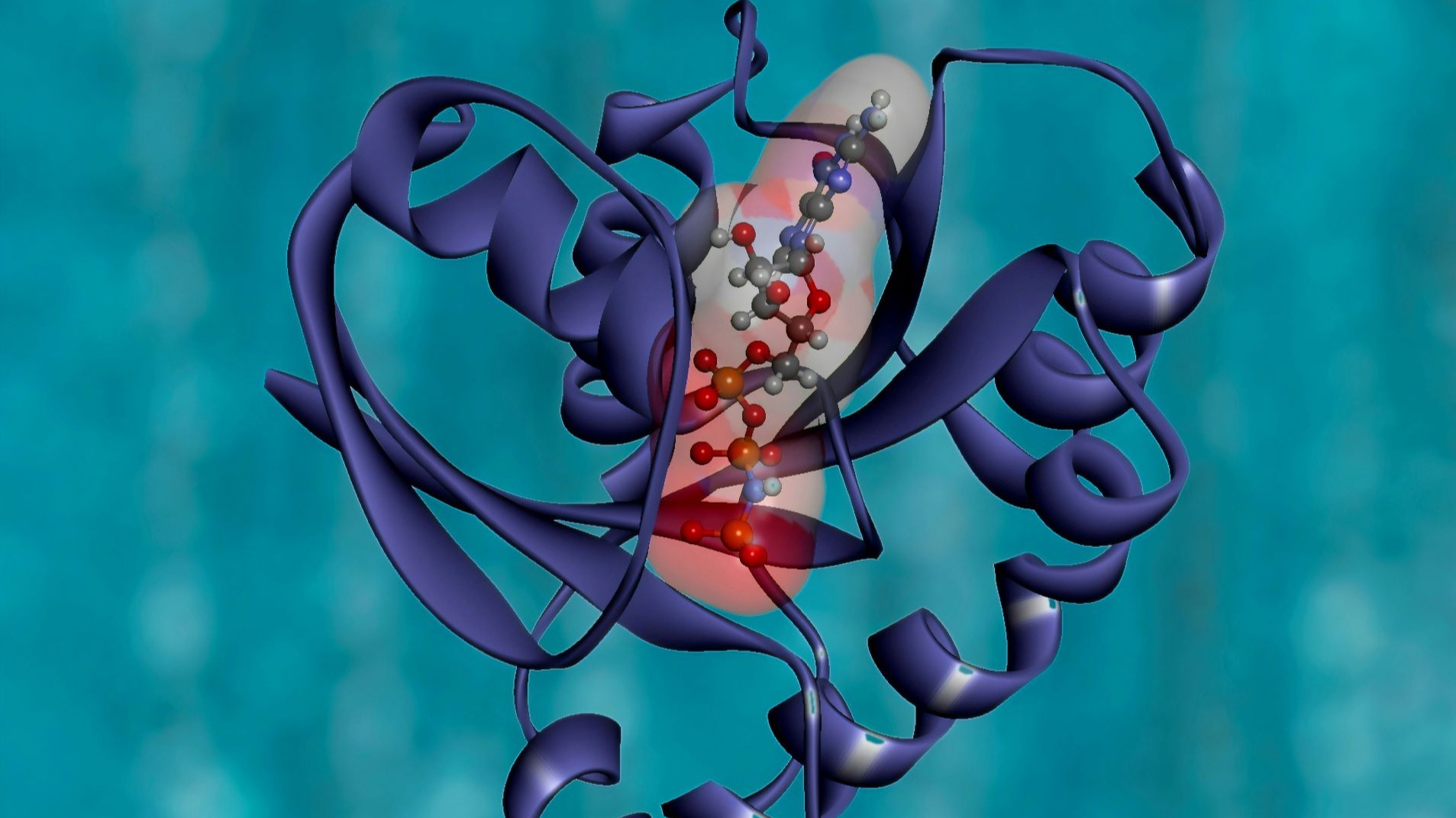
Business Goals
- Cut expenses and duration of early drug discovery phases - target discovery and validation, lead identification and optimization.
- Extend virtual screening capabilities and accuracy by rejecting dubious molecular coordinates and transition to a more efficient parameterization of (bio)molecules.
Challenge
- Experimental methods of measuring binding affinity and inhibition constants are expensive in terms of required human efforts, time, and resources. Due to the tremendous number of chemical compounds, experimental bioactivity screening requires computational approaches.
- There are well-adopted methods of virtual screening, but they either lack generalization capabilities (AutoDock) or are extremely time and computational resources demanding (molecular dynamics).
Results
- The binding affinity estimation MAE is ~1.5 kcal/mol of the Gibbs free energy. Taking into account the energy of water-water hydrogen bond O−H···:O (5.0 kcal/mol), we can conclude that it is a fairly accurate estimate.
- The inference time is 1 ms - not hours, days or weeks as is usually the case in molecular dynamics simulations.
Implementation Details
- We propose a machine learning-based predictor for protein-ligand binding affinities.
- The pipeline unites two subsequent ensembles – classification and regression.
- Within the approach, the binding class and the binding strength can be assessed.
- We show that the use of diverse methods improves the prediction metrics.
Industry
Service
Keywords
- Pharmaceuticals and Biotech
- Molecular Affinity
Roadmap
/*=
$user_is_authed
? declense_numeral(get_field('duration'), 'month', 'months')
: 'X months';
*/ ?>
Solution Design
AI Solutions Architect
Data Collection
Bioinformatician
Exploratory Data Analysis
Bioinformatician
Data Preprocessing
Bioinformatician
Model Development
Bioinformatician
Model Performance Evaluation
Bioinformatician
Hyperparameters Tuning
Bioinformatician
Error Analysis
Bioinformatician
Ensembling Models
Bioinformatician
Release
Sign up to receive the project description
Want to talk?
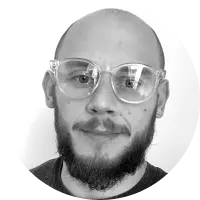
Michael Gurbych
Director,
Operations and Finance
Operations and Finance
Roadmap
/*=
$user_is_authed
? declense_numeral(get_field('duration'), 'month', 'months')
: 'X months';
*/ ?>
Solution Design
AI Solutions Architect
Data Collection
Bioinformatician
Exploratory Data Analysis
Bioinformatician
Data Preprocessing
Bioinformatician
Model Development
Bioinformatician
Model Performance Evaluation
Bioinformatician
Hyperparameters Tuning
Bioinformatician
Error Analysis
Bioinformatician
Ensembling Models
Bioinformatician
Release